目录
独立于任务的 NLP 数据增强
用于 NLP 的 few-shot learning
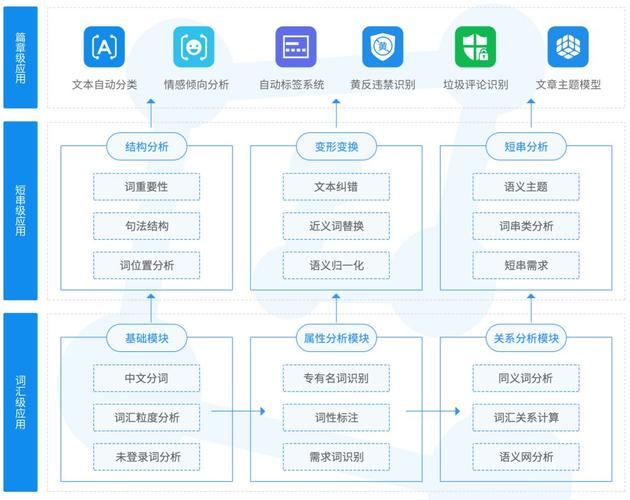
用于 NLP 的的迁移学习
多任务学习
跨措辞学习
独立于任务的架构提升
当开始新领域的研究时,你会创造探求引人瞩目的主题并学会问精确的问题是一件很难的事。这种情形在机器学习这种进展很快的领域里尤其突出——你很难找到打破点。
本文旨在向低级研究职员和试图进入研究领域的人供应启示和思路,个中搜集了我感兴趣的一系列研究课题:着重于自然措辞处理(NLP)和迁移学习,以是本文不是面向所有读者的。如果你对增强学习感兴趣,OpenAI 供应了一系列有趣的增强学习研究主题(https://blog.openai.com/requests-for-research-2/)。如果你期待与他人互助,或对更广泛的主题感兴趣,请参阅 Artificial Intelligence Open Network(https://ai-on.org/)。
这些研究主题中的大多数目前还没有被人们透彻地思考过;在很多情形下,概括性的描述是非常模糊和主不雅观的,未来研究方向也不甚明确。此外,大多数主题也并不包含低挂果实,因此须要我们非常努力才能得出结论。请把稳:这一主题集并不是对付所有文献的综述,以是其覆盖范围可能不全。
希望本文能够引发你的兴趣,并为你自己的研究进程供应灵感。
独立于任务的 NLP 数据增强
数据增强旨在通过转换生产现有演习实例的变体而创建额外的演习数据,以反响现实天下中的实际情形。在打算机视觉中,一样平常的增强技能有镜像、随机裁剪、剪切等。数据增强在 CV 中超级有用,比如有助于 AlexNet 对抗过拟合,绝大多数当前最优模型也利用了它。此外,数据增强非常直不雅观,由于它使得演习数据更加多元,从而提升模型泛化能力。
然而,NLP 中数据增强并未广泛利用。依我看,缘故原由有两点:
1. NLP 中的数据是分离的。这可防止我们把大略的转换直接应用于输入数据。目前绝大多数的增强方法聚焦于这样的转换,比如领域随机化 (Tobin et al., 2017) [2]。
2. 小的扰动可改变语义。删除一个否定词可能会改变句意,修正段落中的一个词可能无意中改变了关于该段落问题的答案。其实在 CV 中情形并非如此:扰动一个像素并不会改变一个猫或狗的图像,并且乃至明显的变革比如不同图像的插值也是有用的(Zhang et al., 2017)[3]。
我关注的现有方法要么是基于规则的 (Li et al., 2017) [5],要么是办理特界说务的,比如解析 (Wang and Eisner, 2016) [6] 或零-代名词分辨率 (Liu et al., 2017) [7]。Xie et al. (2017) [39] 通过来自不同分布的样本替代单词以进行措辞建模和机器翻译。最近聚焦于创建对抗样本的事情要么是通过替代单词或字符 (Samanta and Mehta, 2017; Ebrahimi et al., 2017) [8, 9],级联 (Jia and Liang, 2017) [11];要么是添加对抗扰动 (Yasunaga et al., 2017)。Li et al. (2017) [16] 同样利用了对抗设置,其演习系统生产与人类对话语句无差别的序列。
反向翻译(Back-translation)(Sennrich et al., 2015; Sennrich et al., 2016) [12, 13] 是机器翻译中的常见数据增强方法,有助于接管单语演习数据。比如,当演习一个英转法系统时,单语法语文本通过法转英系统被翻译成英语;合成的平行数据接着被用于演习。反向翻译同样用于释义 (Mallinson et al., 2017) [14]。释义已被用于 QA (Dong et al., 2017) [15] 的数据增强,但并未创造有其他用途。
另一个方法与释义附近,即通过变分自编码器 (Bowman et al., 2016; Guu et al., 2017) [17, 19] 从连续空间中天生语句。如果按照 Hu et al., 2017 [18] 把表征解开,那么我们离风格迁移 (Shen et al., 2017) [20] 也就不远了。
以下几个研究方向很有趣,值得去跟:
1. 评估学习:评估一系列未广泛利用的现有数据增强方法及技巧,比如运用于一系列不同任务(包括文本分类和序列标注)的释义和风格迁移。确定何种类型的数据增强在所有任务和特界说务中是鲁棒的。这可被打装成软件库以使未来的基准更随意马虎。
2. 带有风格迁移的数据增强:调查风格迁移是否可用于修正演习实例的不同属性以得到更鲁棒的学习。
3. 学习增强:类似于 Dong et al. (2017),我们可为一个特界说务学习释义或者天生转换。
4. 学习词嵌入空间以增强数据:范例的词嵌入空间同时聚类同义词和反义词。因此在空间中利用最近邻用于更换是不可行的。受最近事情 (Mrkšić et al., 2017) [21] 启示,我们可以具化词嵌入空间以使其更适用于数据增强。
5. 对抗性数据增强:与最近的可阐明性事情干系 (Ribeiro et al., 2016) [22],我们可以改变实例中最主要的单词,即那些模型依赖以做出预测的单词。但是这依然须要保留语义的更换方法。
用于 NLP 的 Few-shot learning
Zero-shot、one-shot、few-shot learning 是最近最为有趣的研究方向之一。通过屈服 Vinyals et al. (2016) [4] 的核心思想,即 few-shot learning 模型该当明确地演习以实行 few-shot learning,我们已取得多少个最新进展 (Ravi and Larochelle, 2017; Snell et al., 2017) [23, 24]。学习多少个标注样本是最困难的问题之一,以及区分当前机器学习模型天生与更广泛运用的系统的核心能力之一。据我所知,Zero-shot learning 只在学习未知单词的词嵌入的语境中被调查。无数据分类 (Song and Roth, 2014; Song et al., 2016) [25, 26] 是一个有趣的干系方向,它在联合空间中嵌入标签和文件,并须要带有良好描述的可阐明性标签。
1. 标准化基准:为 NLP few-shot learning 创建标准化基准。Vinyals et al. (2016) 为 Penn Treebank 引入了 one-shot 措辞建模任务。这一任务只管很有用,但与 CV 基准上的广泛评估比较却相形见绌,并且据我所知没有多少利用。NLP 的 ew-shot learning 基准该当包含大量分类并供应标准化的再现性分割。良好的候选任务将是主题分类或细粒度实体识别。
2. 评估学习:创建这样一个基准之后,下一步是评估 CV 中的现有 few-shot learning 方法在实行 NLP 任务方面表现如何。
3. NLP 的全新方法:给定一个基准数据集和实证评估学习,接着我们可以开始开拓实行 NLP few-shot learning 的全新方法。
用于 NLP 的迁移学习
迁移学习已经对打算机视觉(CV)产生了巨大的影响,并大大降落理解决特定 CV 问题的难度门槛。打算机视觉从业者现在不再须要为每个新任务耗费大量的工程,仅需利用少量示例对已在大型数据集上演习好的模型进行微调。
然而,在 NLP 领域里,我们目前仍旧只能通过预演习嵌入来预演习模型的第一层。近期一些研究职员提出的方法(Peters et al., 2017, 2018)[31,32] 加入了预演习措辞模型嵌入,但是它们仍旧须要针对每个任务定制架构。在我看来,若想解锁迁移学习在 NLP 上的真正潜力,我们须要预演习全体模型,并在目标任务上仅需微调,类似于微调 ImageNet 模型。举例来说,在 NLP 上的措辞建模可以类比为 CV 上的 ImageNet 分类(Howard and Ruder, 2018)[33]。
这里有一些潜在的研究方向:
1. 识别有用的预演习任务:预演习任务的选择非常主要,纵然是对付干系任务进行微调,我们也可能近会收到有限的成功(Mou et al., 2016)[38]。其他任务,如近期关于学习通用句嵌入的研究(Conneau et al., 2017;Subramanian et al., 2018; Nie et al., 2017)[34,35,40] 可能是措辞模型预演习的补充,或适用于其他目标任务。
2. 繁芜架构的微调:模型运用于多目标时,预演习是最为有效的。然而,目前仍不清楚如何对更为繁芜的模型进行预演习,如用于配对分类任务(Augenstein et al., 2018)或推理任务(如 Q&A 和阅读理解)的模型。
多任务学习
多任务学习(Multi-task learning,MTL)在 NLP 领域中已经变得越来越普遍了。有关多任务学习的概述,可参阅此处(http://ruder.io/multi-task/),有关 NTL 在 NLP 中的目标可参阅此处(http://ruder.io/multi-task-learning-nlp/)。对付我们来说,多任务学习还有很多未知等待我们去探寻。
MTL 的紧张问题带来了一系列有趣的研究方向:
1. 确定有效的赞助任务:个中一个紧张问题是哪些任务对付多任务学习是有用的。标签熵已被证明可以是 MTL 中成功的预测器(Alonso and Plank, 2017)[28],但这一方向并未结束。在最近的研究中(Augenstein et al., 2018)[27],我们创造又跟股东数据和更多细化标签的赞助任务更加有效。未来的 MTL 论文或许不仅会提出新的模型或赞助任务,也会试图开始求解为何很多赞助任务会比另一个紧密干系的任务更好。
2. 硬参数共享的替代方案:硬参数共享目前仍旧是 MTL 的默认操作办法,但它对模型施加了很多约束,以便用相同的参数压缩与不同任务有关的知识,这每每会使学习变得困难。在 MTL 中,我们须要更加易于利用,且在多种任务上事情稳定的新方法(Misra et al., 2017; Ruder et al., 2017)[29,30],标签嵌入层(Augenstein et al., 2018)在这一方向上很有潜力。
3. 人工赞助任务:最好的赞助任务是针对主任务目标,且不须要任何附加数据的任务。在这里,我列出了潜在的人工赞助任务列表(http://ruder.io/multi-task-learning-nlp/)。当然,目前我们还不清楚哪些赞助任务可以在多种不同类型的任务上适用,或哪种基于特界说务的变体性能最好。
跨措辞学习
构建能够跨措辞的模型,将资源丰富措辞中的知识迁移到资源贫乏的措辞中,一贯是 NLP 的主要研究方向之一。最近,学习跨措辞表示,将多种不同措辞投影到共享嵌入空间的方法有了很大进展。可参阅论文《A Survey of Cross-lingual Word Embedding Models》[36]。
跨措辞表示常日根据内部基准测试,或外部下游任务(如文本分类)上的表现进行评估。虽然目前的最前辈方法已经有了很多进步,但我们仍对付这些方法在某些任务或措辞上的失落败缘故原由,以及如何在所有任务上减小这些失落败的方法,如加入基于特界说务的约束(Mrkšić et al., 2017)仍未有足够的理解。
独立于任务的架构提升
目前,在各个特界说务上,业内最佳的成绩正在不断地被刷新,旧的架构正不断被淘汰。之前,我已经列出了在不同 NLP 任务上的最佳实践(http://ruder.io/deep-learning-nlp-best-practices/),但如果不对这些架构在不同任务上的性能进行比较,我们很难定义特定架构的能力,也无法得知它们在其他任务上的可用性。
最近呈现出了一个颇具潜力的模型 Transformer(Vaswani et al., 2017)[37]。虽然完全的模型可能不适用于每个任务,但多头把稳(multi-head attention)或基于位置的编码(position-based encoding)可以作为模块构建模型,这样就可以适用于很多 NLP 任务了。
结论
希望这一研究方向搜集能够对你有所帮助。如果你有关于如何办理干系研究课题的思路,欢迎在本文下进行谈论。
参考文献
1. Krizhevsky, A., Sutskever, I., & Hinton, G. E. (2012). Imagenet classification with deep convolutional neural networks. In Advances in neural information processing systems (pp. 1097-1105).
2. Tobin, J., Fong, R., Ray, A., Schneider, J., Zaremba, W., & Abbeel, P. (2017). Domain Randomization for Transferring Deep Neural Networks from Simulation to the Real World. arXiv Preprint arXiv:1703.06907. Retrieved from http://arxiv.org/abs/1703.06907
3. Zhang, H., Cisse, M., Dauphin, Y. N., & Lopez-Paz, D. (2017). mixup: Beyond Empirical Risk Minimization, 1–11. Retrieved from http://arxiv.org/abs/1710.09412
4. Vinyals, O., Blundell, C., Lillicrap, T., Kavukcuoglu, K., & Wierstra, D. (2016). Matching Networks for One Shot Learning. NIPS 2016. Retrieved from http://arxiv.org/abs/1606.04080
5. Li, Y., Cohn, T., & Baldwin, T. (2017). Robust Training under Linguistic Adversity. In Proceedings of the 15th Conference of the European Chapter of the Association for Computational Linguistics (Vol. 2, pp. 21–27).
6. Wang, D., & Eisner, J. (2016). The Galactic Dependencies Treebanks: Getting More Data by Synthesizing New Languages. Tacl, 4, 491–505. Retrieved from https://www.transacl.org/ojs/index.php/tacl/article/viewFile/917/212%0Ahttps://transacl.org/ojs/index.php/tacl/article/view/917
7. Liu, T., Cui, Y., Yin, Q., Zhang, W., Wang, S., & Hu, G. (2017). Generating and Exploiting Large-scale Pseudo Training Data for Zero Pronoun Resolution. In Proceedings of the 55th Annual Meeting of the Association for Computational Linguistics (pp. 102–111).
8. Samanta, S., & Mehta, S. (2017). Towards Crafting Text Adversarial Samples. arXiv preprint arXiv:1707.02812.
9. Ebrahimi, J., Rao, A., Lowd, D., & Dou, D. (2017). HotFlip: White-Box Adversarial Examples for NLP. Retrieved from http://arxiv.org/abs/1712.06751
10. Yasunaga, M., Kasai, J., & Radev, D. (2017). Robust Multilingual Part-of-Speech Tagging via Adversarial Training. In Proceedings of NAACL 2018. Retrieved from http://arxiv.org/abs/1711.04903
11. Jia, R., & Liang, P. (2017). Adversarial Examples for Evaluating Reading Comprehension Systems. In Proceedings of the 2017 Conference on Empirical Methods in Natural Language Processing.
12. Sennrich, R., Haddow, B., & Birch, A. (2015). Improving neural machine translation models with monolingual data. arXiv preprint arXiv:1511.06709.
13. Sennrich, R., Haddow, B., & Birch, A. (2016). Edinburgh neural machine translation systems for wmt 16. arXiv preprint arXiv:1606.02891.
14. Mallinson, J., Sennrich, R., & Lapata, M. (2017). Paraphrasing revisited with neural machine translation. In Proceedings of the 15th Conference of the European Chapter of the Association for Computational Linguistics: Volume 1, Long Papers (Vol. 1, pp. 881-893).
15. Dong, L., Mallinson, J., Reddy, S., & Lapata, M. (2017). Learning to Paraphrase for Question Answering. In Proceedings of the 2017 Conference on Empirical Methods in Natural Language Processing.
16. Li, J., Monroe, W., Shi, T., Ritter, A., & Jurafsky, D. (2017). Adversarial Learning for Neural Dialogue Generation. In Proceedings of the 2017 Conference on Empirical Methods in Natural Language Processing. Retrieved from http://arxiv.org/abs/1701.06547
17. Bowman, S. R., Vilnis, L., Vinyals, O., Dai, A. M., Jozefowicz, R., & Bengio, S. (2016). Generating Sentences from a Continuous Space. In Proceedings of the 20th SIGNLL Conference on Computational Natural Language Learning (CoNLL). Retrieved from http://arxiv.org/abs/1511.06349
18. Hu, Z., Yang, Z., Liang, X., Salakhutdinov, R., & Xing, E. P. (2017). Toward Controlled Generation of Text. In Proceedings of the 34th International Conference on Machine Learning.
19. Guu, K., Hashimoto, T. B., Oren, Y., & Liang, P. (2017). Generating Sentences by Editing Prototypes.
20. Shen, T., Lei, T., Barzilay, R., & Jaakkola, T. (2017). Style Transfer from Non-Parallel Text by Cross-Alignment. In Advances in Neural Information Processing Systems. Retrieved from http://arxiv.org/abs/1705.09655
21. Mrkšić, N., Vulić, I., Séaghdha, D. Ó., Leviant, I., Reichart, R., Gašić, M., … Young, S. (2017). Semantic Specialisation of Distributional Word Vector Spaces using Monolingual and Cross-Lingual Constraints. TACL. Retrieved from http://arxiv.org/abs/1706.00374
22. Ribeiro, M. T., Singh, S., & Guestrin, C. (2016, August). Why should i trust you?: Explaining the predictions of any classifier. In Proceedings of the 22nd ACM SIGKDD International Conference on Knowledge Discovery and Data Mining (pp. 1135-1144). ACM.
23. Ravi, S., & Larochelle, H. (2017). Optimization as a Model for Few-Shot Learning. In ICLR 2017.
24. Snell, J., Swersky, K., & Zemel, R. S. (2017). Prototypical Networks for Few-shot Learning. In Advances in Neural Information Processing Systems.
25. Song, Y., & Roth, D. (2014). On dataless hierarchical text classification. Proceedings of AAAI, 1579–1585. Retrieved from http://cogcomp.cs.illinois.edu/papers/SongSoRo14.pdf
26. Song, Y., Upadhyay, S., Peng, H., & Roth, D. (2016). Cross-Lingual Dataless Classification for Many Languages. Ijcai, 2901–2907.
27. Augenstein, I., Ruder, S., & Søgaard, A. (2018). Multi-task Learning of Pairwise Sequence Classification Tasks Over Disparate Label Spaces. In Proceedings of NAACL 2018.
28. Alonso, H. M., & Plank, B. (2017). When is multitask learning effective? Multitask learning for semantic sequence prediction under varying data conditions. In EACL. Retrieved from http://arxiv.org/abs/1612.02251
29. Misra, I., Shrivastava, A., Gupta, A., & Hebert, M. (2016). Cross-stitch Networks for Multi-task Learning. In Proceedings of the IEEE Conference on Computer Vision and Pattern Recognition. http://doi.org/10.1109/CVPR.2016.433
30. Ruder, S., Bingel, J., Augenstein, I., & Søgaard, A. (2017). Sluice networks: Learning what to share between loosely related tasks. arXiv preprint arXiv:1705.08142.
31. Peters, M. E., Ammar, W., Bhagavatula, C., & Power, R. (2017). Semi-supervised sequence tagging with bidirectional language models. In Proceedings of the 55th Annual Meeting of the Association for Computational Linguistics (ACL 2017).
32. Peters, M. E., Neumann, M., Iyyer, M., Gardner, M., Clark, C., Lee, K., & Zettlemoyer, L. (2018). Deep contextualized word representations. Proceedings of NAACL.
33. Howard, J., & Ruder, S. (2018). Fine-tuned Language Models for Text Classification. arXiv preprint arXiv:1801.06146.
34. Conneau, A., Kiela, D., Schwenk, H., Barrault, L., & Bordes, A. (2017). Supervised Learning of Universal Sentence Representations from Natural Language Inference Data. In Proceedings of the 2017 Conference on Empirical Methods in Natural Language Processing.
35. Subramanian, S., Trischler, A., Bengio, Y., & Pal, C. J. (2018). Learning General Purpose Distributed Sentence Representations via Large Scale Multi-task Learning. In Proceedings of ICLR 2018.
36. Ruder, S., Vulić, I., & Søgaard, A. (2017). A Survey of Cross-lingual Word Embedding Models. arXiv Preprint arXiv:1706.04902. Retrieved from http://arxiv.org/abs/1706.04902
37. Vaswani, A., Shazeer, N., Parmar, N., Uszkoreit, J., Jones, L., Gomez, A. N., … Polosukhin, I. (2017). Attention Is All You Need. In Advances in Neural Information Processing Systems.
38. Mou, L., Meng, Z., Yan, R., Li, G., Xu, Y., Zhang, L., & Jin, Z. (2016). How Transferable are Neural Networks in NLP Applications? Proceedings of 2016 Conference on Empirical Methods in Natural Language Processing.
39. Xie, Z., Wang, S. I., Li, J., Levy, D., Nie, A., Jurafsky, D., & Ng, A. Y. (2017). Data Noising as Smoothing in Neural Network Language Models. In Proceedings of ICLR 2017.
40. Nie, A., Bennett, E. D., & Goodman, N. D. (2017). DisSent: Sentence Representation Learning from Explicit Discourse Relations. arXiv Preprint arXiv:1710.04334. Retrieved from http://arxiv.org/abs/1710.04334