机器之心编辑部
Composable Kernel(CK)库旨在供应一套在 AMD GPU 上算子领悟的后端方案,该研究希望未来能够移植到 AMD 的所有 GPU 上,并且终极也可以被移植到 AMD CPU 上,该项目已开源。与Meta AITemplate的深度互助大幅提升了AI模型在AMD GPU的端到端性能。
图优化在降落 AI 模型的演习和推理利用的韶光和资源方面起着重要浸染。图优化的一个主要功能是模型中将可以领悟的算子进行领悟,通过降落内存占用和减少数据在低速内存中的搬运来提高打算效率。然而,实现一套能够供应各种算子领悟的后端方案难度很大,导致在实际硬件上 AI 模型能够利用的算子领悟非常有限。
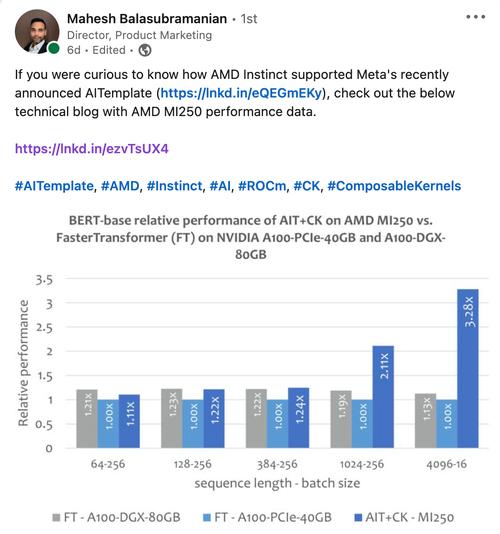
Composable Kernel (CK)库旨在供应一套在 AMD GPU 上的算子领悟的后端方案。CK 利用通用编程措辞 HIP C++,完备开源。其设计理念包括:
高性能 & 高生产力:CK 的核心是一组精心设计,高度优化,可复用的根本模块。CK 库内所有的算子都是通过组合这些根本模块实现的。复用这些根本模块大大缩短开拓后端算法的周期,同时还能担保高性能。精通当前的 AI 问题,快速适应未来的 AI 问题:CK 旨在供应一套完全的 AI 算子后端方案,这让繁芜的算子领悟成为可能,由于这样让全体后端都可以用 CK 实现,而不需依赖外部算子库。CK 的可复用根本模块足以实现常见 AI 模型(机器视觉,自然措辞处理,等等)所需的各种算子及其领悟。当新涌现的 AI 模型须要新的算子时,CK 也将会供应所需的根本模块。AI 系统专家的大略但强大的工具:CK 所有的算子都是用 HIP C++ 模版实现的。AI 系统专家可以通过实例化模版来定制这些算子的属性,比如数据类型,元操作类型,张量存储格式,等等。这常日只须要几行代码。友好的 HIP C++ 界面:HPC 算法开拓者一贯在推动着 AI 打算加速的前沿。CK 的一个主要设计理念便是要让 HPC 算法开拓者更随意马虎对 AI 加速作出贡献。因此 CK 所有核心模块都是用 HIP C++ 实现,而不是 Intermediate Representation (IR)。HPC 算法开拓者直接以他们熟习的编写 C++ 代码的形式编写算法,而无需像基于 IR 的算子库那样,以通过编写针对某种特定算法的 Compiler Pass 来实现。这样做可以大大提高算法的迭代速率。可移植性:本日利用 CK 作为后真个图优化将能够移植到未来 AMD 的所有的 GPU 上,并且终极也可以被移植到 AMD CPU 上【2】。CK 源代码:https://github.com/ROCmSoftwarePlatform/composable_kernel核心观点
CK 引入了两个观点以提高后端开拓者的生产力:
1. 首创性的引入“张量坐标变换” (Tensor Coordinate Transformation)降落 AI 算子的编写繁芜度。该研究首创性地定义了一组可复用的 Tensor Coordinate Transformation 根本模块,并且用它们把繁芜的 AI 算子(比如卷积,group normalization reduction,Depth2Space,等等)以数学严谨的办法重新表达成了最根本的 AI 算子(GEMM,2D reduction,tensor transfer,等等)。这项技能可以让为根本 AI 算子编写的算法直接被用到所有与之对应的繁芜的 AI 算子上,而无需重写算法。
2. 基于 Tile 的编程范式:开拓算子领悟的后端算法可以被算作先将每一个领悟前的算子(独立算子)拆解成许多 “小块” 的数据操作,然后再把这些 “小块” 操作组合成领悟的算子。每一个这样的 “小块” 操作都对应一个原始的独立算子,但是被操作的数据只是原始张量的一部分(tile),因此这样的 “小块” 操作被称为 Tile Tensor Operator。CK 库包含一组针对 Tile Tensor Operator 的高度优化的实现,CK 里所有的 AI 独立算子和领悟算子都是用它们实现的。目前,这些 Tile Tensor Operators 包括 Tile GEMM,Tile Reduction 和 Tile Tensor Transfer。每一个 Tile Tensor Operator 都有针对 GPU thread block,warp 和 thread 的实现。
Tensor Coordinate Transformation 和 Tile Tensor Operator 共同组成了 CK 的可复用的根本模块。
图 1,利用 CK 的 Tensor Coordinate Transformation 根本模块将 convolution 算子表达成 GEMM 算子
图 2,CK 的组成(下:可复用的根本模块;上:独立算子与领悟算子)
代码构造
CK 库构造分为四层,从下到上分别是:Templated Tile Operator,Templated Kernel and Invoker,Instantiated Kernel and Invoker 和 Client API【3】。每一层对应不同的开拓者。
AI 系统专家:“我须要一个后端方案供应高性能的独立和领悟算子让我可以直策应用”。这个例子【4】里用的 Client API 和 Instantiated Kernel and Invoker 供应了预先实例化并编译好的工具,以知足这类开拓者的需求。AI 系统专家:“我为一个开源的 AI 框架做最前辈的图优化事情。我须要一个能够为图优化所需的所有领悟算子供应高性能 kernel 的后端方案。同时我也须要定制这些 kernel,以是像 “要么接管,要么弃用” 的黑盒办理方案不能知足我的需求”。Templated Kernel and Invoker 层能知足这类开拓者。比如这个例子【5】中开拓者可以自己利用 Templated Kernel and Invoker 层实例化出所需的 FP16 的 GEMM + Add + Add + FastGeLU 的 kernel。HPC 算法专家:“我的团队为公司内部不断迭代的 AI 模型开拓高性能后端算法。我们团队中有 HPC 算法专家,但我们仍旧希望可以通过复用和改进硬件供应商供应的高度优化的源代码来提高我们的生产力,并且让我们的代码可以被移植到未来的硬件构架上。我们希望可以不用通过与硬件供应商分享我们的代码来做到这点”。Templated Tile Operator 层可以帮助到这一类开拓者。比如这个代码【6】中开拓者利用 Templated Tile Operator 来实现 GEMM 的优化管线。图 3,CK 库四层构造
基于 AITemplate + CK 的端到端模型推理
Meta 的 AITemplate 【7】(AIT)是一个统一 AMD 和 Nvidia GPU 的 AI 推理系统。AITemplate 利用 CK 作为其 AMD GPU 上的后端,它利用的是 CK 的 Templated Kernel and Invoker 层。
AITemplate + CK 在 AMD Instinct™ MI250 上取得了多个主要 AI 模型最前辈的推理性能。CK 里大多数前辈的领悟算子的定义,都是在 AITemplate 团队的远见下推动的。许多领悟算子的算法也是由 CK 和 AITemplate 团队共同设计。
本文比较了几个端到端模型在 AMD Instinct MI250 和同级别产品【8】的性能表现。本文中所有 AMD Instinct MI250 的 AI 模型的性能数据都是用 AITemplate【9】 + CK【10】取得的。
实验
ResNet-50
下图显示了 AMD Instinct MI250 上的 AIT + CK 与 A100-PCIe-40GB 和 A100-DGX-80GB 上的 TensorRT v8.5.0.12 【11】(TRT)的性能比较。结果显示 AMD Instinct MI250 上的 AIT + CK 取得了比较于 A100-PCIe-40GB 上的 TRT 1.08 倍的加速。
BERT
一个基于 CK 实现的 Batched GEMM + Softmax + GEMM 领悟算子模版,可以完备肃清掉中间结果在 GPU 打算单元(Compute Unit)与 HBM 之间的搬运。通过利用这个领悟算子模版,attention layer 许多原来是带宽瓶颈(bandwidth bound)的问题变成了打算瓶颈(compute bound)的问题,这样可以更好发挥 GPU 的打算能力。这个 CK 的实现深受 FlashAttention 【12】的启示,并比原始的 FlashAttention 的实现减少了更多的数据搬运。
下图显示了 AMD Instinct MI250 上的 AIT + CK 与 A100-PCIe-40GB 和 A100-DGX-80GB 上的 FasterTransformer v5.1.1 bug fix 【13】(FT)的 Bert Base 模型(uncased)的性能比较。当 Sequence 是 4096 时,FT 在 A100-PCIe-40GB 和 A100-DGX-80GB 上会在 Batch 32 时 GPU 内存溢出。因此,在 Sequence 是 4096 时,本文只显示 Batch 16 的结果。结果显示 AMD Instinct MI250 上的 AIT + CK 取得了比较于 A100-PCIe-40GB 上的 FT 3.28 倍,以及比较于 A100-DGX-80GB 上的 FT 2.91 倍的加速。
Vision Transformer (VIT)
下图显示了 AMD Instinct MI250 上的 AIT + CK 与 A100-PCIe-40GB 和 A100-DGX-80GB 上的 TensorRT v8.5.0.12(TRT)的 Vision Transformer Base (224x224 图片)的性能比较。结果显示 AMD Instinct MI250 上的 AIT + CK 取得了比较于 A100-PCIe-40GB 上的 TRT 1.8 倍,以及比较于 A100-DGX-80GB 上的 TRT 1.4 倍的加速。
Stable Diffusion
端到真个 Stable Diffusion
下表显示 AIT + CK 在 AMD Instinct MI250 上 Stable Diffusion 端到端(Batch 1,2,4, 6)的性能数据。当 Batch 是 1 时,在 MI250 上只有一个 GCD 被利用,而在 Batch 2,4,6 时,两个 GCD 都被利用了。
Stable Diffusion 中的 UNet
不过本文还没有关于利用 TensorRT 运行 Stable Diffusion 端到端模型的公开的信息。但这篇文章“Make stable diffusion 25% faster using TensorRT” 【14】解释了怎么利用 TensorRT 加速 Stable Diffusion 中的 UNet 模型。UNet 是 Stable Diffusion 中最主要最花韶光的部分,因此 UNet 的性能大致反应了 Stable Diffusion 的性能。
下图显示了 AMD Instinct MI250 上的 AIT + CK 与 A100-PCIe-40GB 和 A100-DGX-80GB 上的 TensorRT v8.5.0.12(TRT)的 UNet 的性能比较。结果显示 AMD Instinct MI250 上的 AIT + CK 取得了比较于 A100-PCIe-40GB 上的 TRT 2.45 倍,以及比较于 A100-DGX-80GB 上的 TRT 2.03 倍的加速。
更多信息
ROCm webpage: AMD ROCm™ Open Software Platform | AMD
ROCm Information Portal: AMD Documentation - Portal
AMD Instinct Accelerators: AMD Instinct™ Accelerators | AMD
AMD Infinity Hub: AMD Infinity Hub | AMD
Endnotes:
1.Chao Liu is PMTS Software Development Engineer at AMD. Jing Zhang is SMTS Software Development Engineer at AMD. Their postings are their own opinions and may not represent AMD’s positions, strategies, or opinions. Links to third party sites are provided for convenience and unless explicitly stated, AMD is not responsible for the contents of such linked sites and no endorsement is implied. GD-5
2.CK for CPU is in early development phase.
3.C++ APIs for now, Python APIs are under planning.
4.Example of CK “Client API” for GEMM + Add + Add + FastGeLU fused operator. https://github.com/ROCmSoftwarePlatform/composable_kernel/blob/685860c2a9483c9e909d2f8bfb95056672491...
5.Example of CK “Templated Kernel and Invoker” of GEMM + Add + Add + FastGeLU fuse operator. https://github.com/ROCmSoftwarePlatform/composable_kernel/blob/685860c2a9483c9e909d2f8bfb95056672491...
6.Example of using CK “Templated Tile Operator” primitives to write a GEMM pipeline. https://github.com/ROCmSoftwarePlatform/composable_kernel/blob/685860c2a9483c9e909d2f8bfb95056672491...
7.Meta’s AITemplate GitHub repository. https://github.com/facebookincubator/AITemplate
8.MI200-71: Testing Conducted by AMD MLSE 10.23.22 using AITemplate https://github.com/ROCmSoftwarePlatform/AITemplate, commit f940d9b) + Composable Kernel https://github.com/ROCmSoftwarePlatform/composable_kernel, commit 40942b9) with ROCm™5.3 running on 2x AMD EPYC 7713 64-Core Processor server with 4x AMD Instinct MI250 OAM (128 GB HBM2e) 560W GPU with AMD Infinity Fabric™ technology vs. TensorRT v8.5.0.12 and FasterTransformer (v5.1.1 bug fix) with CUDA® 11.8 running on 2x AMD EPYC 7742 64-Core Processor server with 4x Nvidia A100-PCIe-40GB (250W) GPU and TensorRT v8.5.0.12 and FasterTransformer (v5.1.1 bug fix) with CUDA® 11.8 running on 2xAMD EPYC 7742 64-Core Processor server with 8x NVIDIA A100 SXM 80GB (400W) GPU. Server manufacturers may vary configurations, yielding different results. Performance may vary based on factors including use of latest drivers and optimizations.
9.https://github.com/ROCmSoftwarePlatform/AITemplate/tree/f940d9b7ac8b976fba127e2c269dc5b368f30e4e
10.https://github.com/ROCmSoftwarePlatform/composable_kernel/tree/40942b909801dd721769834fc61ad201b5795...
11.TensorRT GitHub repository. https://github.com/NVIDIA/TensorRT
12.FlashAttention: Fast and Memory-Efficient Exact Attention with IO-Awareness. https://arxiv.org/abs/2205.14135
13.FasterTransformer GitHub repository. https://github.com/NVIDIA/FasterTransformer
14.Making stable diffusion 25% faster using TensorRT. https://www.photoroom.com/tech/stable-diffusion-25-percent-faster-and-save-seconds/
15.During their time in AMD
原文链接:https://community.amd.com/t5/instinct-accelerators/amd-composable-kernel-library-efficient-fused-kernels-for-ai/ba-p/553224