信赖(trust)是人类在社会协作中的一种主要的生理状态,人与人之间只有达成了信赖才能更好地展开互助,完成一人无法单独完成的任务。
在人与AI共处的时期,AI同样要与人类建立信赖才能更好地帮助人类。这就哀求AI在两个层次上获取人的信赖:1、能力与性能:AI要让人类清晰地知道在什么条件下,可以完成哪些任务,达到什么样的性能。2、情绪与代价:AI要与人类产生情绪共鸣,保持附近的代价不雅观,把人类的利益放在紧张位置,与人类形成利益共同体。
想象一下,在一个川流不息的十字路口,你乘坐在一辆自动驾驶汽车上,实在是把自己的性命交给了它。当在行驶过程中,它溘然要向左转,但是不见告你为何要向左转,而不是直行或者右转,除非你100%信赖它,否则你很难轻易地接管这个决定。令人遗憾的是,当今的自动驾驶连“能力与性能”的信赖层次都达不到,更不用提“情绪与代价”的信赖层次。这是由于当前基于神经网络的AI算法是有偏见的,可阐明性很差,实质上仍是个“黑盒子”,无法向人类阐明为何做出特定的决策。这一毛病是致命的,尤其是在自动驾驶、金融保险、医疗康健等AI决策能够产生重大影响、风险极高的领域。因此研究可阐明人工智能(XAI)已经势在必行,其目的正是搭建人类和AI之间的信赖桥梁。
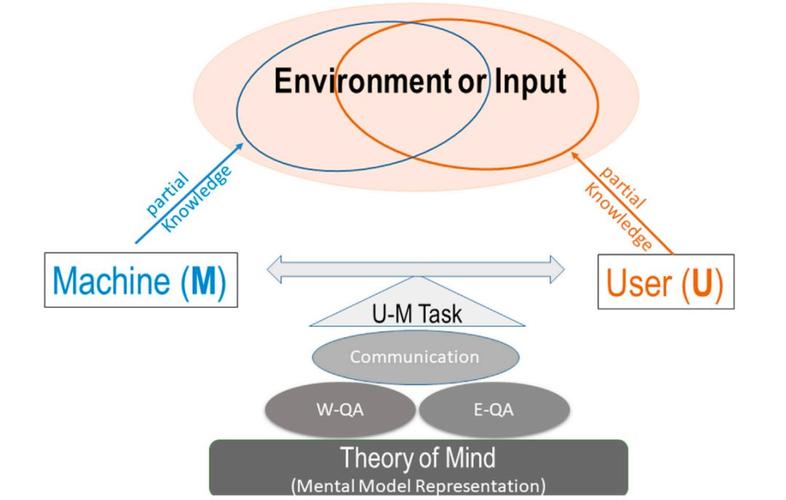
近日,朱松纯教授团队在Cell子刊《iScience》上揭橥了题为《Counterfactual explanations with theory of-mind for enhancing human trust in image recognition models》的论文。
这篇论文提出了一个新的可阐明AI框架CX-ToM,它将“心智理论(ToM)”和“反事实阐明”(counterfactual explanations)集成到了单一的阐明框架中,可以用于阐明深度卷积神经网络(CNN)做出的决策,在图像识别模型中增强人类对神经网络的信赖。
当前可阐明AI框架方向于在单轮对话中天生“阐明”,是一锤子买卖,而本文的一大亮点则是将“阐明”视为一种基于人机交互对话的多轮次通信过程。
图1 CX-ToM论文被Cell子刊《iScience》任命
反事实阐明
心智理论
引用
[1]Agarwal, S., Aggarwal, V., Akula, A.R.,Dasgupta, G.B., and Sridhara, G. (2017). Automatic problem extraction and analysisfrom unstructured text in it tickets. IBM J. Res. Dev. 61, 4–41.
[2]Agarwal, S., Akula, A.R., Dasgupta,G.B., Nadgowda, S.J., Nayak, T.K., 2018. Structured representation andclassification of noisy and unstructured tickets in service delivery. US Patent10,095,779.
[3]Akula, A.R. (2015). A Novel Approachtowards Building a Generic, Portable and Contextual NLIDB System (InternationalInstitute of Information Technology Hyderabad).
[4]Akula, A.R., and Zhu, S.C. (2019).Visual discourse parsing. arXiv, preprint abs/1903.02252. https://arxiv.org/abs/1903.02252.
[5]Akula, A., Sangal, R., and Mamidi, R.(2013). A novel approach towards incorporating context processing capabilitiesin NLIDB system. In Proceedings of the Sixth International Joint Conference onNatural Language Processing, pp. 1216–1222.
[6]Akula, A.R., Dasgupta, G.B., Nayak,T.K., 2018. Analyzing tickets using discourse cues in communication logs. USPatent 10,067,983.
[7]Akula, A.R., Liu, C., Saba-Sadiya, S.,Lu, H., Todorovic, S., Chai, J.Y., and Zhu, S.C. (2019a). X[1]tom:explaining with theory-of-mind for gaining justified human trust. arXiv,preprint arXiv:1909.06907.
[8]Akula, A.R., Liu, C., Todorovic, S.,Chai, J.Y., and Zhu, S.C. (2019b). Explainable AI as collaborative tasksolving. In CVPR Workshops, pp. 91–94.
[9]Akula, A.R., Todorovic, S., Chai, J.Y.,and Zhu, S.C. (2019c). Natural language interaction with explainable AI models.In Proceedings of the IEEE Conference on Computer Vision and PatternRecognition Workshops, pp. 87–90.
[10]Akula, A.R., Gella, S., Al-Onaizan, Y.,Zhu, S.C., and Reddy, S. (2020a). Words aren’t enough, their order matters: onthe robustness of grounding visual referring expressions. arXiv, preprintarXiv:2005.01655.
[11]Akula, A.R., Wang, S., and Zhu, S.(2020b). Cocox: generating conceptual and counterfactual explanations viafault-lines. In The Thirty-Fourth AAAI Conference on Artificial Intelligence,AAAI 2020, the Thirty-Second Innovative Applications of Artificial IntelligenceConference, IAAI 2020, the Tenth AAAI Symposium on Educational Advances inArtificial Intelligence, EAAI 2020 (AAAI Press), pp. 2594–2601.https://aaai.org/ojs/ index.php/AAAI/article/view/5643.
[12]Akula, A., Gella, S., Wang, K., Zhu,S.C., and Reddy, S. (2021a). Mind the context: the impact of contextualizationin neural module networks for grounding visual referring expressions. InProceedings of the 2021 Conference on Empirical Methods in Natural LanguageProcessing, pp. 6398–6416.
[13]Akula, A., Jampani, V., Changpinyo, S.,and Zhu, S.C. (2021b). Robust visual reasoning via language guided neuralmodule networks. Adv. Neural Inf. Process. Syst. 34.
[14]Akula, A.R., Changpinyo, B., Gong, B.,Sharma, P., Zhu, S.C., Soricut, R., 2021c. CrossVQA: scalably generatingbenchmarks for systematically testing VQA generalization.
[15]Akula, A.R., Dasgupta, G.B., Ekambaram,V., Narayanam, R., 2021d. Measuring effective utilization of a servicepractitioner for ticket resolution via a wearable device. US Patent 10,929,264.
[16]Alang, N., 2017. Turns out algorithmsare racist.[online] the new republic.
[17]Alvarez-Melis, D., and Jaakkola, T.S.(2018). On the robustness of interpretability methods. arXiv, preprintarXiv:1806.08049.
[18]Augasta, M.G., and Kathirvalavakumar,T. (2012). Reverse engineering the neural networks for rule extraction inclassification problems. Neural Process. Lett. 35, 131–150.
[19]Bach, S., Binder, A., Montavon, G.,Klauschen, F., Mu¨ ller, K.R., and Samek, W. (2015). On pixel-wise explanationsfor non-linear classifier decisions by layer-wise relevance propagation. PLoSOne 10, e0130140.
[20]Bara, Christian-Paul, Wang, CH-Wang,and Chai, Joyce (2021). Mindcraft: Theory of Mind Modeling for SituatedDialogue in Collaborative Tasks. In Conference on Empirical Methods in NaturalLanguage Processing (EMNLP), 2021 (EMNLP).
[21]Beck, A., and Teboulle, M. (2009). Afast iterative shrinkage-thresholding algorithm for linear inverse problems.SIAM J. Imaging Sci. 2, 183–202.
[22]Berry, D.C., and Broadbent, D.E.(1987). Explanation and verbalization in a computer[1]assisted search task. Q. J.Exp. Psychol. 39, 585–609.
[23]Biran, O., and Cotton, C. (2017).Explanation and justification in machine learning: a survey. In IJCAI-17Workshop on Explainable AI (XAI), p. 1.
[24]Bivens, A., Ramasamy, H., Herger, L.,Rippon, W., Fonseca, C., Pointer, W., Belgodere, B., Cornejo, W., Frissora, M.,Ramakrishna, V. et al., 2017.
[25]Cognitive and contextual analytics for itservices. Bornstein, A.M. (2016). Is artificial intelligence permanentlyinscrutable? Nautilus. Byrne, R.M. (2002).
[26]Mental models and counterfactual thoughtsabout what might have been. Trends Cogn. Sci. 6, 426–431. Byrne, R.M. (2017).
[27]Counterfactual thinking: from logic tomorality. Curr. Dir. Psychol. Sci. 26, 314–322. Champlin, C., Bell, D., andSchocken, C. (2017).
[28]AI medicine comes to Africa’s ruralclinics. IEEE Spectr. 54, 42–48. Chancey, E.T., Bliss, J.P., Proaps, A.B., andMadhavan, P. (2015).
[29]The role of trust as a mediator betweensystem characteristics and response behaviors. Hum. Factors 57, 947–958. Clark,H.H., and Schaefer, E.F. (1989). Contributing to discourse. Cogn. Sci. 13,259–294.
[30]Clark, K., Khandelwal, U., Levy, O.,and Manning, C.D. (2019). What does Bert look at? An analysis of Bert’sattention. arXiv, preprint arXiv:1906.04341.