本文实例讲述了PHP实现机器学习之朴素贝叶斯算法。分享给大家供大家参考,详细如下:
机器学习已经在我们的生活中变获得处可见了。比如从你在家的时候温控器开始事情到智能汽车以及我们口袋中的智好手机。机器学习看上去已经无处不在并且是一个非常值得探索的领域。但是什么是机器学习呢?常日来说,机器学习便是让系统不断的学习并且对新的问题进行预测。从大略的预测购物商品到繁芜的数字助理预测。
在这篇文章我将会利用朴素贝叶斯算法Clasifier作为一个类来先容。这是一个大略易于履行的算法,并且可给出满意的结果。但是这个算法是须要一点统计学的知识去理解的。在文章的末了部分你可以看到一些实例代码,乃至自己去考试测验着自己做一下你的机器学习
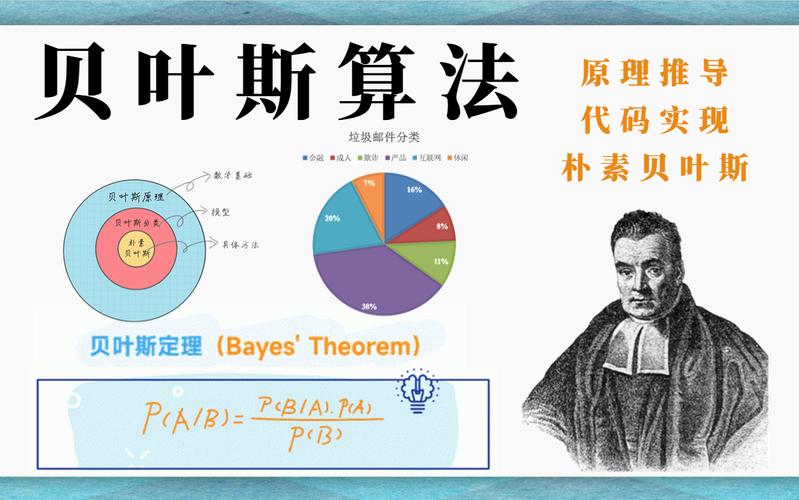
起步那么,这个Classifier是要用来实现什么功能呢?实在它紧张是用来判断给定的语句是积极地还是悲观的。比如,“Symfony is the best”是一个积极的语句,“No Symfony is bad”是一个悲观的语句。以是在给定了一个语句之后,我想让这个Classifier在我不给定一个新的规则的情形就返回一个语句类型。
我给Classifier命名了一个相同名称的类,并且包含一个guess方法。这个方法接管一个语句的输入,并且会反回这个语句是积极的还是悲观的。这个类就像下面这样:
| class Classifier| {| public function guess($statement)| {}| }我更喜好利用列举类型的类而不是字符串作为我的返回值。我将这个列举类型的类命名为Type,并且包含两个常量:一个POSITIVE,一个NEGATIVE。这两个常量将会当做guess方法的返回值。
| class Type| {| const POSITIVE = 'positive';| const NEGATIVE = 'negative';| }初始化事情已经完成,接下来便是要编写我们的算法进行预测了。
朴素贝叶斯
朴素贝叶斯算法是基于一个演习凑集事情的,根据这个演习集从而做出相应的预测。这个算法利用了大略的统计学以及一点数学去进行结果的打算。比如像下面四个文本组成的演习凑集:
如果给定语句是“Symfony is the best”,那么你可以说这个语句是积极地。你平常也会根据之前学习到的相应知识做出对应的决定,朴素贝叶斯算法也是同样的道理:它根据之前的演习集来决定哪一个类型更加附近。
学习
在这个算法正式事情之前,它须要大量的历史信息作为演习集。它须要知道两件事:每一个类型对应的词产生了多少次和每一个语句对应的类型是什么。我们在履行的时候会将这两种信息存储在两个数组当中。一个数组包含每一类型的词语统计,另一个数组包含每一个类型的语句统计。所有的其他信息都可以从这两个数组中聚合。代码就像下面的一样:
| function learn($statement, $type)| {| $words = $this->getWords($statement);| foreach ($words as $word) {| if (!isset($this->words[$type][$word])) {| $this->words[$type][$word] = 0;| }| $this->words[$type][$word]++; // 增加类型的词语统计| }| $this->documents[$type]++; // 增加类型的语句统计| }有了这个凑集往后,现在这个算法就可以根据历史数据接管预测演习了。
定义
为理解释这个算法是如何事情的,几个定义是必要的。首先,让我们定义一下输入的语句是给定类型中的一个的概率。这个将会表示为P(Type)。它因此已知类型的数据的类型作为分子,还有全体演习集的数据数量作为分母来得出的。一个数据便是全体演习集中的一个。到现在为止,这个方法可以将会命名为totalP,像下面这样:
| function totalP($type)| {| return ($this->documents[$type] + 1) / (array_sum($this->documents) + 1);| }请把稳,在这里分子和分母都加了1。这是为了避免分子和分母都为0的情形。
根据上面的演习集的例子,积极和悲观的类型都会得出0.6的概率。每中类型的数据都是2个,一共是4个数据以是便是(2+1)/(4+1)。
第二个要定义的是对付给定的一个词是属于哪个确定类型的概率。这个我们定义成P(word,Type)。首先我们要得到一个词在演习集中给出确定类型涌现的次数,然后用这个结果来除以全体给定类型数据的词数。这个方法我们定义为p:
| function p($word, $type)| {| $count = isset($this->words[$type][$word]) ? $this->words[$type][$word] : 0;| return ($count + 1) / (array_sum($this->words[$type]) + 1);| }在本次的演习集中,“is”的是积极类型的概率为0.375。这个词在全体积极的数据中的7个词中占了两次,以是结果便是(2+1)/(7+1)。
末了,这个算法该当只关心关键词而忽略其他的成分。一个大略的方法便是将给定的字符串中的单词分离出来:
| function getWords($string)| {| return preg_split('/\s+/', preg_replace('/[^A-Za-z0-9\s]/', '', strtolower($string)));| }准备事情都做好了,开始真正履行我们的操持吧!
预测
为了预测语句的类型,这个算法该当打算所给定语句的两个类型的概率。像上面一样,我们定义一个P(Type,sentence)。得出概率高的类型将会是Classifier类中算法返回的结果。
为了打算P(Type,sentence),算法当中将用到贝叶斯定理。算法像这样被定义:P(Type,sentence)= P(Type) P(sentence,Type)/ P(sentence)。这意味着给定语句的类型概率和给定类型语句概率除以语句的概率的结果是相同的。
那么算法在打算每一个相同语句的P(Tyoe,sentence),P(sentence)是保持一样的。这意味着算法就可以省略其他成分,我们只须要关心最高的概率而不是实际的值。打算就像这样:P(Type,sentence) = P(Type) P(sentence,Type)。
末了,为了打算P(sentence,Type),我们可以为语句中的每个词添加一条链式规则。以是在一条语句中如果有n个词的话,它将会和P(word_1,Type) P(word_2,Type) P(word_3,Type) .....P(word_n,Type)是一样的。每一个词打算结果的概率利用了我们前面看到的定义。
好了,所有的都说完了,是时候在php中实际操作一下了:| function guess($statement)| {| $words = $this->getWords($statement); // 得到单词| $best_likelihood = 0;| $best_type = null;| foreach ($this->types as $type) {| $likelihood = $this->pTotal($type); //打算 P(Type)| foreach ($words as $word) {| $likelihood = $this->p($word, $type); // 打算 P(word, Type)| }| if ($likelihood > $best_likelihood) {| $best_likelihood = $likelihood;| $best_type = $type;| }| }| return $best_type;| }
这便是所有的事情,现在算法可以预测语句的类型了。你要做的便是让你的算法开始学习:| $classifier = new Classifier();| $classifier->learn('Symfony is the best', Type::POSITIVE);| $classifier->learn('PhpStorm is great', Type::POSITIVE);| $classifier->learn('Iltar complains a lot', Type::NEGATIVE);| $classifier->learn('No Symfony is bad', Type::NEGATIVE);| var_dump($classifier->guess('Symfony is great')); // string(8) "positive"| var_dump($classifier->guess('I complain a lot')); // string(8) "negative"github上完全php代码如下:| <?php| class Type| {| const POSITIVE = 'positive';| const NEGATIVE = 'negative';| }| class Classifier| {| private $types = [Type::POSITIVE, Type::NEGATIVE];| private $words = [Type::POSITIVE => [], Type::NEGATIVE => []];| private $documents = [Type::POSITIVE => 0, Type::NEGATIVE => 0];| public function guess($statement)| {| $words = $this->getWords($statement); // get the words| $best_likelihood = 0;| $best_type = null;| foreach ($this->types as $type) {| $likelihood = $this->pTotal($type); // calculate P(Type)| foreach ($words as $word) {| $likelihood = $this->p($word, $type); // calculate P(word, Type)| }| if ($likelihood > $best_likelihood) {| $best_likelihood = $likelihood;| $best_type = $type;| }| }| return $best_type;| }| public function learn($statement, $type)| {| $words = $this->getWords($statement);| foreach ($words as $word) {| if (!isset($this->words[$type][$word])) {| $this->words[$type][$word] = 0;| }| $this->words[$type][$word]++; // increment the word count for the type| }| $this->documents[$type]++; // increment the document count for the type| }| public function p($word, $type)| {| $count = 0;| if (isset($this->words[$type][$word])) {| $count = $this->words[$type][$word];| }| return ($count + 1) / (array_sum($this->words[$type]) + 1);| }| public function pTotal($type)| {| return ($this->documents[$type] + 1) / (array_sum($this->documents) + 1);| }| public function getWords($string)| {| return preg_split('/\s+/', preg_replace('/[^A-Za-z0-9\s]/', '', strtolower($string)));| }| }| $classifier = new Classifier();| $classifier->learn('Symfony is the best', Type::POSITIVE);| $classifier->learn('PhpStorm is great', Type::POSITIVE);| $classifier->learn('Iltar complains a lot', Type::NEGATIVE);| $classifier->learn('No Symfony is bad', Type::NEGATIVE);| var_dump($classifier->guess('Symfony is great')); // string(8) "positive"| var_dump($classifier->guess('I complain a lot')); // string(8) "negative"结束语只管我们只进行了很少的演习,但是算法还是该当能给出相瞄准确的结果。在真实环境,你可以让机器学习成百上千的记录,这样就可以给出更精准的结果。